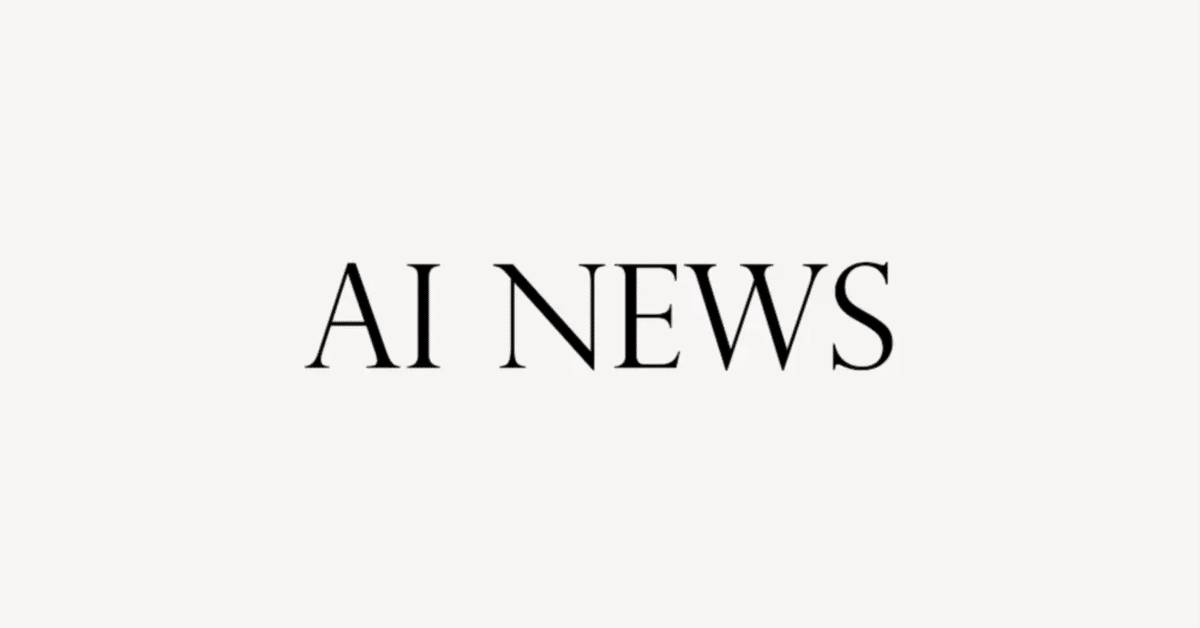
【AIの光と影:進化する技術の社会的影響】英語解説を日本語で読む【2024年2月1日|@David Shapiro】
この動画はAIに関するアンケート結果と最新の研究を基に、AIの現状と未来について解説しています。AIの進歩は速まり、法律、医療、科学研究などの分野で効率化とコスト削減が期待されています。例えば、法律分野ではAIによる契約書作成や調査でコストが大幅に削減される見込みです。医療と科学研究でも、AIの導入により迅速かつ正確な結果が期待されています。しかし、一部の職業はAIにより代替される危機に直面しています。それでも、AIは新たな機会をもたらし、より高度な業務への集中が可能になるとされています。総じて、AIの進歩は多大な効率化とコスト削減をもたらし、同時に職業の未来に影響を与えると予想されます。
公開日:2024年2月1日
※動画を再生してから読むのがオススメです。
As a creator and communicator in the topic of AI, my perspective is a little bit different from the audiences.
AIの話題でクリエイターやコミュニケーターとして、私の視点は聴衆とは少し異なるものです。
And so, I ran this poll and it was actually kind of surprising.
そこで、このアンケートを実施しましたが、実際には驚くべき結果でした。
I said, How are you feeling about AI overall? Which emotion is strongest?
「AI全体についてどう感じていますか?最も強い感情は何ですか?」と聞きました。
Lost confused was pretty small.
迷惑や混乱はかなり少なかったです。
Worried and anxious was a little bit bigger.
心配や不安は少し大きかったです。
Excited and enthusiastic was far and away the biggest one.
興奮と熱意が圧倒的に最も大きかったです。
And then tepid cautious, and then other.
そして、それから冷淡で用心深く、その他と続きます。
And then a bunch of people had some really hilarious comments, saying, You know, being torn between enthusiastic and worried.
そして、多くの人々が本当に面白いコメントをしていて、「熱狂と心配の間で葛藤している」と言っていました。
All of the above was kind of a common one.
「上記全て」というのも一般的な回答でした。
Some people were apathetic, some people like, Whatever, it's overhyped, which that's fair.
一部の人々は無関心で、一部の人々は「どうでもいい、過大評価されている」と言っていました。それは公平ですね。
But if the people that had that as their response, that was in the five percent.
しかし、そのような回答をした人々は5%に過ぎませんでした。
So, it seems that the strongest emotion is excitement and enthusiasm for AI.
したがって、最も強い感情はAIに対する興奮と熱意のようです。
So, this is a good reality check for me.
これは私にとって良い現実的なチェックです。
And the reason that I want to explain this, and that it's, I promise that you'll find this interesting and valuable information, is because, I mean, you know me, I'm always super optimistic, but I also recognize that that is an emotional bias of mine.
そして、私がこれを説明したい理由、そしてそれが興味深く価値のある情報であることを約束する理由は、私は常に非常に楽観的ですが、それは私の感情的な偏見だと認識しているからです。
And when I say emotional bias, I just mean that, like, even in spite of the problems that I see, I also see a path forward.
感情的な偏見と言うと、私が見る問題にもかかわらず、私は解決策の道を見出しているという意味です。
And so, hope and optimism are kind of like the opposite of, I don't know, maybe cynicism, because if you feel like there's problems and there's no solution, then you'd have to be crazy to be optimistic.
そして、希望と楽観主義は、疑念とは逆のようなものです。なぜなら、問題があって解決策がないと感じるなら、楽観的であるためには狂っている必要があるからです。
You know, in terms of, like, problems, like one of the biggest ones that's coming up is, like misinformation, disinformation.
問題の一つとして、誤情報やディスインフォメーションが出てくるということです。
There was the whole Taylor Swift thing, which I'm not going to talk about because it's kind of, kind of, kind of gross, kind of nasty.
テイラー・スウィフトの件がありましたが、それについては話さないでおきます。なぜなら、それはちょっと、ちょっと、ちょっと嫌な話だからです。
If you really want to know, go to Reddit.
本当に知りたい場合は、Redditに行ってください。
They'll tell you all about the Taylor Swift thing.
彼らがテイラー・スウィフトのことをすべて教えてくれます。
Anyways, so what I did want to do was, like, let's check in and I'll kind of walk you through because, because the reason it's that tension between problems, yes, but also a path forward.
とにかく、私がしたかったのは、チェックインして、あなたに歩きながら説明することです。問題という緊張感と、進むべき道との間にある緊張感の理由です。
And so, there are problems, but there's also, at the same time, evidence of the path forward.
問題はありますが、同時に進むべき道の証拠もあります。
Before we launch into the rest of the video, I had a question from a patreon supporter, which, by the way, thank you.
ビデオの残りの部分に入る前に、パトロンのサポーターからの質問がありました。ちなみに、ありがとうございます。
Some of my best questions and best videos are prompted by my patreon supporters, so keep them coming.
私の最高の質問や最高の動画のいくつかは、私のパトロンのサポーターからの提案によるものですので、引き続き提案をお願いします。
The question basically, the world, I'll tell you more about the answer because the question led to this answer.
基本的に質問は、世界についてです。質問がこの回答につながったので、もっと詳しく回答します。
But the question was more or less like, Okay, well, where are we at in terms of takeoff or in terms of launch or whatever?
しかし、質問はおおよそ、「では、離陸や開始の段階はどのくらい進んでいますか?」というようなものでした。
And I said, We are very, very obviously and clearly at the knee of the curve.
私は「私たちは明らかに曲線の膝にいる」と言いました。
And so, what I mean by the knee of the curve is back here?
膝の曲線とは、ここに戻ることを意味しますか?
This is 2015, 2016, 2018, where yes, there was progress, but it was few and far between and it also was not immediately exciting or impactful.
これは2015年、2016年、2018年で、進展はあったものの、まばらで即座に興奮や影響を与えるものではありませんでした。
And now, here we are and everyone has been joking for the last six months, like, Oh, we get AI breaking news on a daily or hourly basis.
そして、ここにいる今、過去6か月間、皆が冗談を言っていました。「AIの最新ニュースは毎日または毎時報告されています」。
But it's still not changing the world.
しかし、それでも世界は変わっていません。
And another thing that's happening is that the Overton window is shifting.
そして、もう一つ起こっていることは、オーバートンウィンドウが変化していることです。
And so, I'll talk about that in just a second.
それでは、すぐにそのことについて話します。
And so, the pace of AI is very obviously faster today than it was even two years ago, even a full year and a half ago, a year ago.
そして、AIのペースは、明らかに今日の方が2年前、1年半前、1年前よりも速くなっています。
We had ChatGPT, so that's kind of when we really entered the knee of the curve.
ChatGPTが登場したのはその頃で、それが曲線の膝に本当に入った時期です。
But we're also not where we want to be for those of us that are excited about the future and the acceleration.
しかし、私たちが将来と加速に興奮している私たちにとっては、まだ望んでいる場所にはいません。
And so, if this slow takeoff here was like the last five years and this knee of the curve is like the last year, and then that's why it's like I'm still pretty confident that by September 2024, if many more of you might be convinced about the AGI thing, I don't know, who knows?
したがって、この遅いスタートが過去5年間であり、この曲線の膝が過去1年間であり、それから2024年9月までに、もっと多くの人々がAGIのことに納得する可能性があるということです。誰にもわかりませんが。
There was a recent talk or interview Sam Altman has been going on a lot of interviews.
最近、サム・アルトマンがたくさんのインタビューを受けているという話がありました。
And I think it was him who said that, like, or maybe someone else talked about how competitive dynamics might force all the big AI companies to go faster, to accelerate their release window.
そして、競争力のあるダイナミクスがすべての大手AI企業をより速く進めるように迫るかもしれない、と彼が言ったのは彼だったと思います。リリースウィンドウを加速させるために。
And so, I have a whole other video planned on, like, competitive dynamics and Red Queen Theory.
そして、私は競争力のある動態とレッドクイーン理論について別のビデオを計画しています。
But after seeing the polls, I think you guys, like, you get it.
しかし、アンケートを見た後、あなたたちは理解してくれているようです。
So, I don't need to beat the dead horse on that one.
だから、それについてはもう話す必要はありません。
You guys are excited and you're with me.
あなたたちは興奮していて、私と一緒です。
And so, what I want to point out is that by the end of this year and certainly by 2025, by 12 months from now, we should probably be feeling like we're entering into this more vertical phase, this higher point of acceleration.
私が指摘したいのは、今年の終わりまで、そして確実に2025年までに、私たちはより垂直なフェーズに入っているように感じるはずだということです。加速の高い地点です。
In part because of those competitive dynamics, in part because of compounding returns, and I'll talk about why that is technically about to happen in just a moment.
競争力のある動態の一部、複利のリターンの一部によるものです。そして、なぜそれが技術的に起ころうとしているのかについて、ちょっとした説明をします。
So, now to throw a bucket of cold water on it, this is why it's like, I see this and it's like, you might say ostensibly it's bad news, but I say it's actually kind of a mixed blessing.
では、冷水をかけることにしましょう。これが、私が見ると、表面的には悪いニュースかもしれませんが、実際には一種の幸運だと言える理由です。
So, everyone has been talking about all the tech layoffs happening.
皆がテック企業の大量解雇について話しています。
And of course, like, if you look on Reddit, you look on other places, translators, copywriters, marketing people, all kinds of people are being laid off or put on notice because of AI.
もちろん、Redditや他の場所を見れば、翻訳者、コピーライター、マーケティング担当者など、さまざまな人々がAIの影響で解雇されたり、注意を促されたりしています。
And so, what I will say is this is nothing new.
そして、私が言いたいのは、これは何も新しいことではないということです。
So, I've worked for a bunch of big tech companies.
だから、私はいくつかの大手テック企業で働いてきました。
The biggest one was Cisco Systems in Silicon Valley.
最大のものはシリコンバレーのCisco Systemsでした。
And so, every time the technology landscape changes, tech companies go through huge layoffs.
そして、技術の風景が変わるたびに、テック企業は大規模な人員削減を経験します。
When all the tech companies were pivoting to the age of the internet, when they're pivoting to virtualization, when they're pivoting to software as a service, all of these things cause layoffs as companies pivot.
インターネット時代にテック企業が転換する際、仮想化に転換する際、ソフトウェアサービスに転換する際、これらすべてが企業の転換に伴って人員削減を引き起こします。
And so, the way that it was explained to me is this is just part of the business.
そして、私に説明されたのは、これは単にビジネスの一部であるということです。
Because as the organization, as the company, and the bigger they are, the more branches they have, and it's kind of like if you ever go through the forest and you see like trees drop off branches that are no longer useful, like that's actually a thing that happens.
なぜなら、組織や企業として、そしてそれが大きければ大きいほど、枝が多くなるため、森を歩いていると、もはや役に立たない枝が落ちていくのを見ることがあります。それが実際に起こることなのです。
It's called dieback.
それは「枯死」と呼ばれます。
And like, this is companies going through the same process.
そして、これは企業が同じプロセスを経ているのです。
It's like, Oh, hey, there's no sunlight over here anymore.
まるで、「ここにはもう日光がないな」という感じです。
So let me get rid of that branch and put a new branch out where there is sunlight.
だから、その枝を取り除いて、日光のある場所に新しい枝を出そうとするのです。
So, some of this is just par for the course as technology evolves and shifts.
だから、これは技術が進化し変化するにつれて当然のことの一部です。
Now, one thing that remains to be seen, though, is are these layoffs permanent?
ただし、まだわからないことが一つあります。それは、これらの人員削減が永続的なものなのかということです。
Because there's evidence to suggest that, like, hey, this actually dislocates a lot of workers.
なぜなら、これによって多くの労働者が不安定な状況になるという証拠があるからです。
It invalidates the need for some workers.
一部の労働者の必要性が無効化されるのです。
And of course, people like me predict that that's only going to accelerate.
そしてもちろん、私のような人々は、これが加速するだけだと予測しています。
However, it looks like right now, you do still really need a human in the driver's seat.
ただし、現時点では、運転席には人間が本当に必要です。
But that if you have these tools, these AI and automation tools that allow one person to get the work done of ten people, remember, that's what I did as an automation engineer, that absolutely reduces headcount.
しかし、これらのツール、つまりAIと自動化のツールがあれば、1人で10人分の仕事をこなすことができるので、それは確かに人員削減につながります。
This is a double-edged sword because on the one hand, yes, it sucks if people are getting laid off.
これは二律背反の剣です。一方では、人々が解雇されるのは悲しいことです。
But on the other hand, if people are getting laid off because AI is ready for prime time, that means AI is ready for prime time.
しかし、もう一方では、AIが本格的に使えるようになったために人々が解雇されるのであれば、それはAIが本格的に使えるようになったということです。
That's what we wanted, right?
それが私たちが望んだことですよね?
And so, it's kind of like, be careful what you wish for.
だから、望むべきことには注意が必要です。
There's always a double-edged sword to some of these things.
これらのことには常に二律背反の剣があるのです。
And so, now I'll end on kind of the main news that I wanted to get to that kind of inspired this whole thing.
そして、今回の話をインスパイアしたメインのニュースで終わります。
So, every now and then, I'll go through the archive and look for the latest and greatest papers.
時々、私はアーカイブを調べて、最新かつ最高の論文を探します。
When I do that, sometimes it's just a bunch of incremental improvements.
それをすると、時にはただの改善の一環であることもあります。
Sometimes it's a bunch of little algorithmic things, which, yes, I'm glad they're happening, but they're not necessarily exciting to write home about.
時にはいくつかの小さなアルゴリズム的なことがあります。それは嬉しいことですが、家族に報告するほど興奮することではありません。
But this one was really kind of caught my attention.
しかし、これは本当に私の注意を引きました。
And so, it's better called GPT, comparing Large Language Models against lawyers.
そして、それはGPTと呼ばれるもので、弁護士との比較において大規模な言語モデルに対抗するものです。
So, I've got some lawyer friends who are in the tech space.
私にはテック業界にいる弁護士の友人がいます。
We talk about this kind of stuff and I, as soon as I saw this, I sent them, send it to them and they kind of know that it's coming.
私たちはこのようなことについて話し合っており、私がこれを見た瞬間、彼らに送りました。彼らはこれが起こることを知っています。
So, I've consulted with a bunch of lawyers who just say yeah, they see the writing is on the wall.
私はいくつかの弁護士と相談しましたが、彼らはAIについて学び、それに参入するつもりだと言っています。
They're gonna learn about AI and they're gonna, they're gonna get in it. And so, let's just zoom in on the most salient point, cost-wise.
彼らはAIについて学び、それに参加することになるでしょう。そして、最も重要なポイントであるコストに焦点を当てましょう。
LLMs operate at a fraction of the price, offering a staggering 99.97 percent reduction in cost, reduction in cost over traditional methods.
大規模な言語モデルは、従来の方法に比べてコストが99.97%削減されるという驚異的なものです。
Now, what I want to point out is this is for evaluating like basic contracts like NDAs.
今、指摘したいのは、これはNDAなどの基本的な契約の評価に対してのものです。
But when something costs 99.97 percent less than a human, that goes to that.
しかし、何かが人間の99.97%安い価格で提供されるということは、それに該当します。
You know, better, faster, cheaper, safer.
より良く、より速く、より安く、より安全です。
And what you'll see in the data of this is that in many cases, it is at parity with humans.
そして、このデータでは、多くの場合、人間と同等の結果が得られることがわかります。
And especially once you're using like GPT-4 11..., the preview.
特に、GPT-4 11...のプレビューを使用しているときは、その点を強調したいですね。
So, I'm not gonna go through all of the details of the paper.
紙の詳細をすべて説明するつもりはありません。
Suffice to say, I read most of it.
言うまでもなく、私はほとんど読みました。
I skimmed the rest.
残りはざっと目を通しました。
And they did a pretty, pretty good job of saying, okay, let's have humans from America and New Zealand do this, which have slightly different legal systems.
そして、彼らはアメリカとニュージーランドの人々にこれを行わせることをかなりうまくやったと言えます。これらの国々はわずかに異なる法制度を持っています。
And what they found was that the LLM actually did generalize pretty well across different legal systems.
そして彼らが見つけたのは、大規模言語モデルが実際に異なる法制度においてかなりうまく一般化されたということです。
And so, the reason that that's important is because you have nations that are case law and then you have nations that are statutory law.
そして、それが重要な理由は、判例法の国と法令法の国があるからです。
Which basically just means if it's very common law, common law is when you have where you have precedent.
基本的には、非常に一般的な法律であれば、判例法が適用されます。
And so in America when you hear the news like so-and-so, the argument of Stanley versus Tucker versus 1837, when you have to refer to precedent, that is common law or case law, as I mistakenly said.
そして、アメリカでは、ニュースで「スタンリー対タッカー対1837年」といったように、判例を参照する必要がある場合、それは判例法またはケースローと呼ばれます。私が間違って言ったように。
Which is basically that you look at least at established legal precedent as part of the legal process.
基本的には、法的プロセスの一環として確立された法的先例を少なくとも参照するということです。
And then in statutory nations, only the letter of the law matters.
そして、法令法の国では、法律の文字通りのみが重要です。
Prior precedent doesn't matter.
先例は重要ではありません。
And then in America, we actually have kind of a hybrid system, which I just learned.
そして、アメリカでは、実際にはハイブリッドなシステムがあります。私はつい最近知りました。
Anyway, so this is why lawyers are wizards.
とにかく、これが弁護士が魔法使いである理由です。
This is because when you have to one, understand that every state has slightly different approaches.
これは、すべての州がわずかに異なるアプローチを持っていることを理解しなければならないからです。
And then, every specialization within the legal system also has different norms and standards.
そして、法制度内のすべての専門分野には異なる規範と基準があります。
And then, there are literally just mountains of text to go through.
そして、文字通り山ほどのテキストを読まなければなりません。
But that is why AI is ideally suited to do this.
しかし、それがAIがこれを行うのに理想的に適している理由です。
And of course, there's a legal service called case text which my lawyer friends already use and they're like, yeah, this basically replaces a paralegal.
もちろん、すでに弁護士の友人たちが使っているケーステキストという法的サービスがあります。これは実質的にパラリーガルを置き換えるものです。
It does the work of several paralegals and it does it faster.
それは数人のパラリーガルの仕事をこなし、それをより速く行います。
Now you might say, like, alright.
さて、あなたは言うかもしれません、よし。
Well, this is ostensibly bad news for lawyers, but what I want to talk about is why.
まあ、これは明らかに弁護士にとっては悪いニュースですが、私が話したいのはなぜかということです。
One, if AI is ready to reduce costs by 99.97%, which obviously that's not the full story.
まず、AIがコストを99.97%削減できる準備ができているということです。もちろん、それは全体の話ではありませんが。
That's just doing one task, but still.
それはただ1つのタスクを行うだけですが、それでも十分価値があります。
If it's ready to do that in something as complex as law and it can do it at near human, near or above human performance, but it's that much cheaper, it's worth it.
もし法律のような複雑なものでも、ほぼ人間と同等、またはそれ以上の性能でできるなら、それは非常に安価なので、それは価値があります。
And so I use AI extensively in my thing.
私は自分の仕事でAIを広範に使用しています。
It's why I'm able to do this solo now.
それが私が今独りでこれを行うことができる理由です。
I use AI editors, I use AI to help do the writing and so on and so forth.
AIエディターを使用し、執筆を手伝ってもらっています。
Obviously this one I'm just kind of riffing.
もちろん、今回はちょっとした即興です。
But you know, so here where I say like do it with NDAs anyways. So if AI is at that point, yes, it means that we are at risk for disrupting the status quo.
でもね、ここで私が「とにかくNDAでやってください」と言っているのは、AIがそのポイントに達しているならば、それは私たちの現状を変えるリスクがあるということです。
But it also means that AI is ready.
しかし、それはまた、AIが準備ができているということでもあります。
AI is at this point.
AIはこの段階にあるのです。
And if it can help with law, then it can help with medicine and it can help with scientific research because you know, you can we kind of always say like doctors and lawyers.
そして、それが法律に役立つのであれば、医学や科学的研究にも役立つことができます。医者や弁護士のように、高給で高生産性のある、希少なスキルセットとして見なされるものですから。
Doctors and lawyers are kind of held up as like the pinnacle of high-paid, highly productive, rarefied skill sets. And you know, there's also been some studies out there showing that AI is at or above most human doctors, most physicians in terms of offering rapid diagnosis.
医師や弁護士は、高給与で高生産性であり、希少なスキルセットの頂点として見なされています。また、AIは多くの研究で、迅速な診断を提供する点で、ほとんどの人間の医師、ほとんどの医師よりも優れていることが示されています。
But again, if it costs 1% of the price or in this case, 0.03% of the price, because they basically said let me get to get down to the data.
しかし、もし価格が1%、またはこの場合は0.03%しかかからないのであれば、それは価値があります。なぜなら、AIはほぼ人間と同等以上のパフォーマンスでそれを行うことができるからです。
Let's see.
見てみましょう。
Where was it?
さて、どこだったかな。
This was accuracy.
これは正確さのことです。
They hear here they look at the loss and so you see the LPO, the kind of senior-grade lawyer, GPT-4 32k was almost as good as a senior partner.
彼らはここで聞いて、損失を見て、LPO(法律事務所の上級弁護士)として、GPT-4 32kは上級パートナーとほぼ同じくらい優れていました。
So that's that.
それがそれです。
And then on this one, it was even closer, so the GPT-4 1106.
そして、これに関しては、さらに近くなりました。GPT-4 1106は。
But then you go down to the time.
しかし、時間を見てみましょう。
So the senior lawyer took 43 minutes on average, junior lawyer 56 minutes, LPO 200 minutes on average.
上級弁護士は平均して43分かかり、初級弁護士は56分、LPOは平均して200分かかりました。
And then GPT-4 took less than five minutes on average.
そして、GPT-4は平均して5分以下かかりました。
So it's ten times faster than even, almost ten times, eight times faster than a senior lawyer.
それによって、シニア弁護士の約10倍の速さで動作します。
So that's a lot of cognitive load that's been taken off.
それはかなりの認知負荷が軽減されたことを意味します。
And then if you saw Wes Roth video and other people's video talking about Microsoft's future of work, this is pretty well established now that what these tools allow you to do is perform a lot of cognitive offload.
そして、もしウェス・ロスのビデオや他の人々のビデオでMicrosoftの未来の仕事について話しているのを見たなら、これはかなり確立されていることです。これらのツールによって、多くの認知オフロードが可能になります。
But then you look at the cost.
しかし、コストを見てみましょう。
So senior lawyers are very expensive and so in this case the average was $76, which okay, $76 for three-quarters of an hour.
上級弁護士は非常に高価ですので、この場合の平均は76ドルでした。まあ、3/4時間で76ドルです。
So that means they're billing out at $100 an hour.
つまり、彼らの請求額は1時間あたり100ドルです。
That's honestly relatively cheap.
それは正直に言って比較的安いです。
Like, I mean some lawyers bill out at five hundred, six hundred, eight hundred dollars an hour.
例えば、一部の弁護士は1時間に500ドル、600ドル、800ドルと請求しています。
So depending on the contract, now you probably wouldn't have someone ultra high-powered looking over an NDA.
契約によりますが、おそらく超高額の弁護士がNDAを見直すことはないでしょう。
But my point is that okay, so even for a relatively low-paid lawyer in the grand scheme of things, I don't want to say like oh, this is a cheapo, but I know lawyers that charge a lot more than this.
しかし、私のポイントは、相対的に低給与の弁護士でも、全体的な観点から見れば、これよりもずっと高い料金を請求する弁護士がいるということです。
And so this amount of labor on average was about $76 and the cost for some of these was much, much lower.
したがって、この労働量の平均は約76ドルであり、これらのいくつかのコストははるかに低かったです。
Whoops.
おっと。
No, don't submit it.
いや、送信しないでください。
That's nothing wrong with it.
それには何も問題はありません。
So GPT-4, the cost of tokens was 25 cents.
GPT-4、トークンのコストは25セントでした。
And so you have it's literally, let's see, what is that?
つまり、それは文字通り、さて、何ですか?
300 times cheaper if my mental math is correct.
もし私の頭の計算が正しければ、300倍安いです。
When you have something that is 300 times cheaper than a human and it's at near parity.
人間の300倍も安く、ほぼ同等の性能を持つものがあるとき、それは重要です。
So because remember, look at the loss and so I actually had that wrong.
だから、覚えておいてください、損失を見て、実際に私はそれを間違えていました。
Like legal professional, so that's not a senior partner anyways.
法律の専門家のように、それはとにかく上級パートナーではありません。
But the point is that we don't have mental models for this level of cost disruption.
しかし、ポイントは、このレベルのコストの混乱についての心のモデルを持っていないことです。
The best that we can talk about is 200 years ago, 95% of people were farmers and now only 2% of people are farmers.
私たちが話すことができる最高のことは、200年前には95%の人々が農民であり、今ではわずか2%の人々が農民であるということです。
That is the level of disruption we're looking at now.
それが私たちが今見ている混乱のレベルです。
This also underscores the possibility of Jevons paradox.
これはまた、ジェヴォンズの逆説の可能性を強調しています。
So Jevons paradox basically says that as the cost of something goes down, as the efficiency of that resource goes up, you actually sometimes paradoxically end up with more use of it.
ジェヴォンズの逆説は、何かのコストが下がるにつれて、そのリソースの効率が上がると、実際には時には逆説的にそれをより多く使用することになるということを基本的に述べています。
And so this is basic supply and demand.
そして、これは基本的な供給と需要です。
So as supply goes up, cost goes down, therefore utilization goes up.
供給が増えると、コストが下がり、したがって利用が増えます。
Now in some cases, the unmet demand of a resource is basically infinite.
一部の場合、リソースの未満の需要は基本的に無限です。
So what I mean by that is that electricity is an example.
それが何を意味するかというと、電気がその例です。
The cheaper we can make electricity, the more we use it.
電気を安くするほど、それをより多く使用します。
Now you might say there, another example is as the cost of fuel goes down, people drive more.
さて、そこで言えるのは、もう1つの例として、燃料のコストが下がると、人々はより多く運転するということです。
Yeah, but people are not going to be driving 24-7.
そうですが、人々は24時間365日運転するわけではありません。
There's still a finite plateau of demand for fuel and automotive services.
燃料と自動車サービスの需要にはまだ有限の平坦地があります。
Now you might also say, but yeah, what if you could fly around the world for $20?
さて、あなたはまた言うかもしれませんが、そうですね、もし20ドルで世界中を飛び回れるとしたらどうでしょうか?
Would people fly more?
人々はより多く飛ぶでしょうか?
Yeah, it's actually possible.
そうです、実際に可能です。
But even then, some people would fly more, some people wouldn't.
しかし、それでも、一部の人々はより多く飛ぶかもしれませんが、一部の人々はそうではないかもしれません。
But electricity is the kind of thing that scales almost logarithmically, which is why you have the Kardashev scale.
しかし、電気はほぼ対数的にスケールするものであり、それがなぜカルダシェフスケールがあるのかです。
Right now, we're still like a what, a point six on the Kardashev scale because we can imagine uses for more and more electricity.
現在、私たちはまだカルダシェフスケールの0.6のようなものです。なぜなら、私たちはますます多くの電気の使用方法を想像できるからです。
So like once we get to nuclear fusion, that will be like we can recycle all the landfills, we can recycle all the lithium, we can clean up the atmosphere, like there's so much that we can do just at a Kardashev One scale.
だから、核融合に到達したら、それはまるで私たちがすべての埋立地をリサイクルできるようになり、すべてのリチウムをリサイクルできるようになり、大気をきれいにすることができるようになるかのようです。カルダシェフスケールの1つのスケールでできることはたくさんあります。
I don't know that there's going to be much beyond that, but then again, that could be a limitation of my imagination.
それ以上のことはわかりませんが、それは私の想像力の制限かもしれません。
But the point is that our ability to produce, consume, and use energy seems to scale almost indefinitely.
しかし、ポイントは、私たちのエネルギーを生産し、消費し、使用する能力はほぼ無限にスケールするようです。
So Jevons paradox definitely applies there.
ですから、ジェヴォンズの逆説はそこに確かに適用されます。
But the question is, does Jevons paradox apply to other things like legal services and medical services and scientific services?
しかし、問題は、ジェヴォンズの逆説が法律サービスや医療サービス、科学サービスなどの他のものにも適用されるかどうかです。
I think that it does apply to science because again, the more you know about science, the more you know that we know almost nothing.
私はそれが科学に適用されると思います。なぜなら、科学について知っていることが多ければ多いほど、私たちがほとんど何も知らないことがわかるからです。
We have mountains and mountains of research and centuries of research behind us, but it's just like, yeah, we hardly know anything.
私たちは山ほどの研究と何世紀もの研究を背負っていますが、実際には、私たちはほとんど何も知りません。
That's one of the most common refrains when I talk to any PhD or Postdoc.
それは私がどんな博士号や博士研究員と話すときに最もよく聞く言葉の一つです。
I'm like what are you an expert in?
私はあなたは何に詳しいのですか?
And they're just like, I don't know anything.
そして彼らはただ、何も知らないと言うだけです。
Like, yeah, we know a lot, but compared to what we don't know, yeah.
まあ、私たちはたくさん知っていますが、知らないことと比べると、そうですね。
So science, that seems to be logarithmically scaling where there's just basically no limit to how much science we need to do.
だから、科学は対数的にスケールするようで、私たちが行う科学の量には基本的に制限がありません。
So the cheaper and faster and better we can do science, the more science we're gonna get done.
だから、私たちが科学をより安く、より速く、より良く行えるほど、私たちはより多くの科学を行うことができます。
So that's one of the things that I'm most excited about when I see a report like this.
だから、私がこのような報告書を見たときに最も興奮することの一つです。
It's like, oh, hey, if we can reduce the cost of science by 300, by a factor of 300, not 300 percent, by 300 X, that would be great.
まるで、ああ、もし私たちが科学のコストを300分の1に減らすことができたら、それは素晴らしいことです。
Now, I don't know that we're gonna see the same commensurate rise in demand for legal services.
さて、法的サービスの需要の同等の上昇を見ることができるかどうかはわかりません。
I don't know about you, but like, I only need legal services every now.
あなたはわかりませんが、私はたまにしか法的サービスは必要ありません。
And then, but one thing that I predicted in one of my first books on AI, which is outdated so you don't even bother go reading it, but is that if you can do more legal stuff faster, then you're gonna see more legal stuff being done, particularly in businesses and government.
その後、私がAIについての最初の本の一つで予測したことですが、その本は古くなってしまったので読む必要はありませんが、もしあなたがより多くの法的なことをより速く行うことができるなら、特にビジネスや政府の分野で、より多くの法的なことが行われるようになるでしょう。
And this is, I honestly suspect that Biden's executive order up on AI, the longest executive order in history.
そして、これは、正直に言って、バイデン大統領のAIに関する行政命令、史上最長の行政命令だと疑っています。
I don't know if they wrote it with AI, but I'm pretty sure that they reviewed it with AI, which is one of the reasons that it was so big and so legal wrangling either between companies or within nations or between nations.
彼らがAIでそれを書いたかどうかはわかりませんが、AIでそれをレビューしたことはほぼ確実であり、それが企業間や国内外、国家間の法的な争いの一因であると思われます。
I suspect that one of the downstream effects is that this is going to have a pretty sizable impact on diplomacy, negotiations, and treaty because it allows for translation.
私は、これが外交、交渉、条約にかなりの影響を与えると予想しています。なぜなら、これによって翻訳が可能になるからです。
It allows for more sophisticated agreements and that sort of thing.
より洗練された合意が可能になりますし、そのようなことも可能です。
And yeah contract negotiation is a big thing.
そして、契約交渉は大きな問題です。
One of the attorneys that reached out to me to consult back when we only had GPT-3, that was actually his specialty was contract negotiation, and contract negotiation is something that can take years and cost millions of dollars.
私たちがGPT-3しか持っていなかった時に、私に相談するために連絡を取ってきた弁護士の一人は、実際には契約交渉が専門でした。契約交渉は数年かかり、数百万ドルの費用がかかることもあります。
But what if, instead of taking years and costing millions of dollars, it takes a couple days and costs ten thousand dollars?
しかし、数年かかり、数百万ドルの費用がかかる代わりに、数日かかり、1万ドルの費用がかかるとしたらどうでしょうか?
No matter how big the contract negotiation is, or that is what we're getting close to now.
契約交渉がどれほど大きくても、それが今に近づいているのです。
Of course, for you and me, for your average Joe, like we don't really care because we're not involved in those multi-million or multi-billion dollar contract negotiations.
もちろん、あなたや私、一般の人々にとっては、私たちは数百万ドルや数十億ドルの契約交渉に関与していないので、それほど気にする必要はありません。
But if the same level of cognitive labor can be put towards healthcare, towards medical research, towards material science research, at the same rate and at the same cost, that is going to be that compounding return that we're hoping to see, and that is going to get us closer to getting past the knee of the curve and more into the fun part of the curve.
もし同じレベルの認知的労働が、医療、医学研究、材料科学研究に向けられ、同じ速度で、同じコストで行われるなら、それは我々が望んでいる複利のようなリターンを生み出すことになるでしょう。そして、それは私たちをカーブの膝を越えることに近づけ、より楽しい部分のカーブに入ることになるでしょう。
I think that's what I'll call this.
これを私はそう呼ぶと思います。
This is, we're at the knee and so boring, knee fun.
これは、私たちが曲線の膝にいて、退屈な部分ですが、楽しい部分が来るということです。
Alright, so anyways, that's, that's thanks for chiming in on the excitement and so on and so forth.
とにかく、それが、興奮やその他のことについてのご意見をいただき、ありがとうございます。
So, cheers.
それでは、乾杯です。
Have a good day.
良い一日を。
この記事が気に入ったらサポートをしてみませんか?