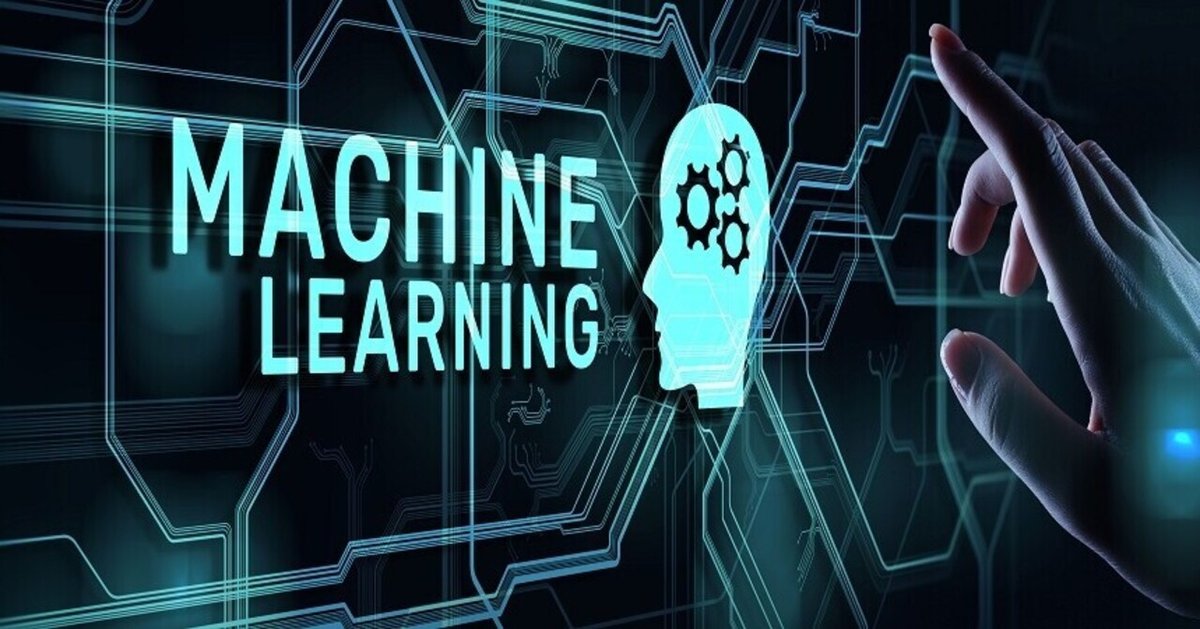
What Is Machine Learning? Types Of ML and Uses
Machine learning (ML) is the use of computing that enables you to enforce and improve a framework without being ambiguous. Machine learning is dynamic and does not rely on coded and rule-based instructions with the best machine learning Training course in Delhi.
Machine Learning Applications
Web Search - Ranking page supports what you can click on.
Computer Biology - Medicine in Rational Style on Laptop Supports Past Experiments.
Finance - Decide to whom Mastercard offers should be sent. Risk analysis for loan offers. how to decide where to speculate with cash.
Electronic commerce - predicting the loss of customers. whether a business is dishonourable or not.
Space exploration - domestic probes and astronomy.
Robotics - the way to face uncertainty in new environments. Autonomous. Autonomous car.
Information extraction - query databases across the entire network.
Social networks - information about relationships and preferences. Machine learning to extract prices from information.
Debugging - Used in engineering topics such as debugging. Labor intensive method. it can be recommended as long as the error is.
Types of Machine Learning Problems
Fallback: For fallback problems, performance is consistent.
Sequence: Here an event or a category of the data in a predefined packet is anticipated. The return could be a defined value.
Clustering: In this edition we tend to characterize the data in n categories, if n is not predefined. The highlight of the arrangement issue is that the number of meetings is not predetermined; for example, the grouping of buyers in comparative meetings passionate about their socio-economic aspects, interests and history.
Machine Learning: Algorithms and Types
Supervised Learning
With managed learning, the calculus learns about the mentioned data set, wherever the reaction is understood. This, as "director", is about organizing the model that provides a solution key that the computation uses to evaluate its accuracy in providing data. This can be used to anticipate qualities for future or hidden dates. This can be a strategy driven by the assignment of the associate title. Cases of buyer enthusiasm from the supervised study area, constant loss of personnel, identification of deception, evaluation of the commercial value of things, anticipation of membership refunds, spam segregation, handwriting confirmation.
Semi-supervised learning
Semi-managed learning is between managed and unsupported learning processes. It uses both named and unlabelled dates for preparation where named dates are often a modest amount, and unlabelled dates are also gigantic in variety. Useful for speech research, online page grouping, caption, text documentation.
Unsupervised learning
In individual learning, the model assumes the key construction, the style of the unlabelled data. There is no response and no gift of the target variable in such data to conduct the research, of course, what is right is not right. The machine tries to recognize the instance and delivers the response. Although there is no trace of the best performance, the data is sorted or broken down by grouping. The calculation shows how to effectively distinguish between the essence of a person and the substance of a pony or a cat. this can be a behaviour-driven process. Examples of unsupported learning: Buyers Division, Image Division, Market Inquiry, Business Promotion, Characteristic Clumsy Regions.
Reinforced learning
Supportive learning depends on the critique group wherever there is a specialist and a prepared climate. This strategy is behavioural and depends on attachments learned through experimentation. Here the specialist finds the way to act in a very favourable climate, enjoying certain activities and noting the prices and results that he obtains when creating these movements. An example of this can be how to ride a motorcycle. This method will improve the useful efficiency of frames, as well as mechanical technology, scholasticism, coordination of production networks and manufacturing.
Uses of Machine Learning
One of the conflicting problems with AI is that this set of strategies is used everywhere. It is widely used in enterprises and fields. Some of the models are Quora specific response ratings used in search queries, wellness trackers like Fitbits. The uses of machine learning are:
Speech recognition: It is an associate degree interaction to change the language suggestions and search in a message. It is the interpretation of words expressed verbally in the text. Completely different remote helpers - for example, Google Home Speakers, Amazon's Alexa, Google Assistant, Apple's Siri, Microsoft's Cortana - use this strategy.
Programmed language translation, auto-correcting: The machines interpret text that begins with one language and then the following spelling errors.
Expectation: Millilitres can be used to anticipate and assume completely different perspectives, such as inventory forecasts, travel times, weather, store business, prepaid.
Image confirmation: abuse of image sharing strategies, machines recognize objects, people, places, extended cinematographic material.
Suggestion Engines: This area unit used by all web-based retailers, internet movie apps to view the next article or internet movie or series, depending on whether they received or read a story from the person and they chose to get excited. what others have bought or seen excited about your call.
Clinical findings: Machine learning can be compensated to distinguish between incurable and non-fatal illness.
Money transactions and exchanges: companies use to distinguish exchanges and fake customers, to create credit checks and delinquent loans, to improve exchange
この記事が気に入ったらサポートをしてみませんか?