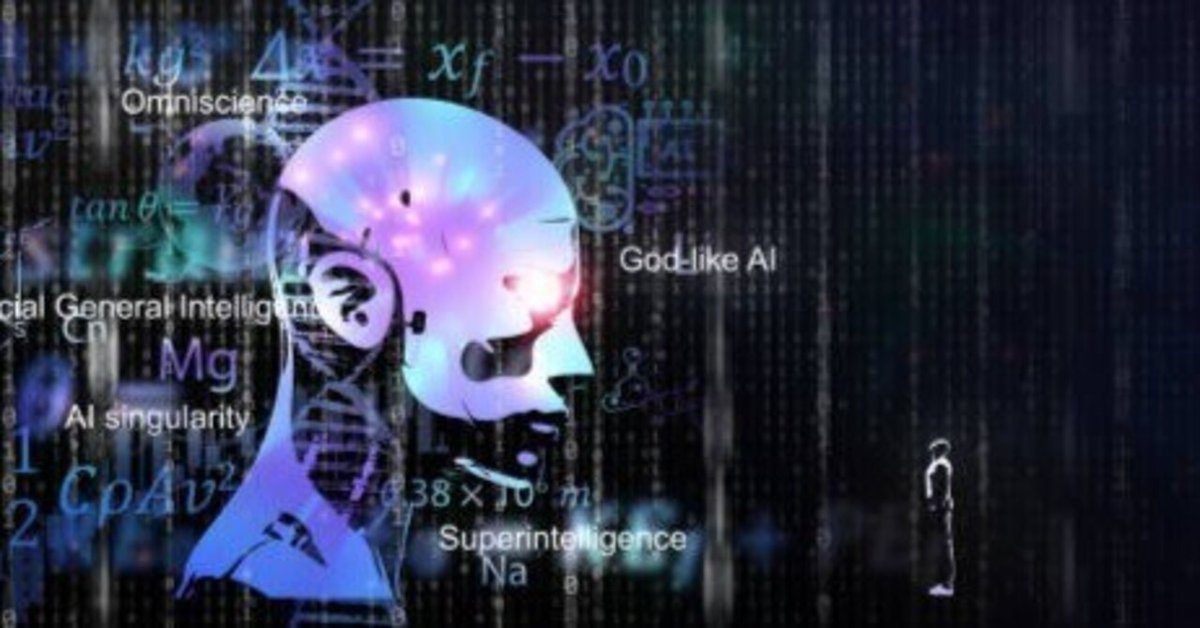
【ChatGPT】日本語プロンプトと英語プロンプトで出力結果に差が出るか検証してみた
検証の背景
日本語の学習データ量に比べ英語の学習データ量が非常に多いため、学習データ量の違いによる生成AIの出力結果に質と量の差を見る
ChatGPTバージョン
GPT-4o
使用プロンプト
以下日本語と英語のプロンプトを使用しました
■日本語
人工知能の倫理的課題について、具体的な例を挙げながら詳細に説明してください。
■英語
Please explain the ethical issues related to artificial intelligence, providing specific examples in detail.
出力結果
日本語プロンプトによる出力
人工知能(AI)の倫理的課題は多岐にわたります。以下に具体的な例を挙げて、詳細に説明します。
### 1. 偏見と差別
**具体例:** AIによる採用プロセスの自動化
AIが履歴書のスクリーニングを行うシステムを導入した企業では、過去の採用データに基づいてモデルを訓練します。もしそのデータが特定の性別、年齢、人種に対して偏っている場合、AIはその偏見を学習してしまい、特定のグループを不当に排除する可能性があります。これにより、差別が強化され、平等な機会が損なわれるリスクがあります。
### 2. プライバシーの侵害
**具体例:** 顔認識技術の使用
顔認識技術は防犯や利便性の向上に役立つ一方で、個人のプライバシーを侵害する危険性もあります。例えば、公共の場や商業施設での監視カメラに顔認識技術が導入されると、個人の行動が常に監視されることになり、プライバシーの侵害や監視社会の到来が懸念されます。また、このデータが不正に利用されたり、悪用されたりするリスクもあります。
### 3. 説明可能性の欠如
**具体例:** 医療診断システム
AIを用いた医療診断システムは、病気の早期発見や診断の精度向上に寄与しますが、その判断の根拠が不明確な場合があります。例えば、AIが特定の診断を下す際に、医師や患者に対してその判断基準を明示できないと、診断結果の信頼性が疑問視される可能性があります。この説明可能性の欠如は、医療過誤のリスクを高めるとともに、医師と患者の信頼関係を損なう恐れがあります。
### 4. 自律兵器の倫理的問題
**具体例:** ドローンによる攻撃
自律型の軍事ドローンは、敵の標的を自動で検出し、攻撃を行う能力を持つことができます。しかし、これには重大な倫理的課題が伴います。人間の判断を介さずに攻撃を行うことで、誤ったターゲットを攻撃するリスクが高まり、無実の民間人が犠牲になる可能性があります。また、戦争の道徳的な側面が軽視され、人命の軽視が進む危険性があります。
### 5. 労働市場への影響
**具体例:** ロボットの導入による雇用喪失
製造業やサービス業において、AIやロボットの導入が進むと、多くの人々が仕事を失う可能性があります。例えば、自動運転車の普及はタクシードライバーやトラック運転手の仕事を脅かします。このような技術の進歩が社会全体にどのような影響を与えるのかを慎重に考える必要があります。労働市場の変動に伴う再教育や社会保障の充実が求められます。
### まとめ
AIの倫理的課題は、技術の発展とともにますます重要なテーマとなっています。偏見の排除、プライバシーの保護、説明可能性の確保、自律兵器の規制、労働市場の影響への対処など、多岐にわたる問題に対して、慎重かつ包括的なアプローチが求められます。これにより、AI技術が社会にとって有益で公正なものとなるように努める必要があります。
英語プロンプトによる出力
Artificial intelligence (AI) presents a range of ethical issues that span privacy, bias, accountability, and transparency. Here are some specific examples illustrating these ethical concerns in detail:
### Privacy
**Example: Facial Recognition Technology**
Facial recognition systems, such as those used by law enforcement or in public surveillance, can infringe on individuals’ privacy. These systems can track and identify people without their consent, leading to a surveillance state where individuals are constantly monitored. This can be particularly invasive when used in public spaces or for tracking individuals’ movements over time.
### Bias and Fairness
**Example: Hiring Algorithms**
AI systems used in hiring, like Amazon’s now-discontinued recruiting tool, have shown biases against certain groups. Amazon’s tool favored male candidates because it was trained on resumes submitted over a 10-year period, which were predominantly from men. This led to the algorithm penalizing resumes that included terms associated with women, such as “women’s chess club captain.”
**Example: Predictive Policing**
Predictive policing algorithms, like those used in some U.S. cities, can perpetuate and exacerbate existing biases in law enforcement. These systems often rely on historical crime data, which can be biased against minority communities. As a result, these communities may be unfairly targeted and over-policed.
### Accountability
**Example: Autonomous Vehicles**
When autonomous vehicles, like those developed by Tesla or Waymo, are involved in accidents, determining accountability can be challenging. If an AI-driven car causes a collision, it’s unclear who is responsible: the manufacturer, the software developer, or the user? This lack of clear accountability can complicate legal and insurance frameworks.
**Example: AI in Healthcare**
AI systems used in healthcare for diagnostics or treatment recommendations, such as IBM’s Watson for Oncology, can make errors. When an AI system misdiagnoses a patient or suggests an incorrect treatment, determining who is accountable for the mistake — the AI developers, the medical practitioners, or the healthcare providers — becomes complex.
### Transparency
**Example: Credit Scoring Algorithms**
Credit scoring algorithms, such as those used by FICO, often operate as black boxes, making it difficult for individuals to understand why they received a particular score. This lack of transparency can lead to unfair lending practices and make it challenging for individuals to contest or improve their scores.
**Example: Social Media Algorithms**
Platforms like Facebook and YouTube use complex algorithms to curate content for users. These algorithms prioritize engagement, sometimes promoting sensationalist or misleading content to keep users on the platform longer. The lack of transparency about how these algorithms work and what data they use can manipulate public opinion and contribute to the spread of misinformation.
### Ethical Use of AI in Warfare
**Example: Autonomous Weapons**
The development of autonomous weapons, such as drones that can identify and engage targets without human intervention, raises significant ethical concerns. These weapons can make life-and-death decisions without human oversight, potentially leading to unintended casualties or escalation of conflicts. The use of AI in warfare also poses the risk of lowering the threshold for entering armed conflicts, as it reduces the immediate human cost of war.
### Impact on Employment
**Example: Automation in Manufacturing**
AI and automation technologies, such as those used in manufacturing by companies like Foxconn, can lead to significant job displacement. While these technologies can increase efficiency and reduce costs, they also replace human workers, leading to unemployment and social inequality. The ethical challenge lies in balancing technological advancement with the need to protect and retrain the workforce.
### Ethical AI Development
**Example: Google’s Project Maven**
Google’s involvement in Project Maven, a Pentagon initiative to use AI for analyzing drone footage, sparked ethical concerns among employees and the public. The project raised questions about the ethical implications of using AI for military purposes and led to internal protests at Google, resulting in the company deciding not to renew its contract with the Department of Defense.
### Conclusion
Addressing these ethical issues requires a multifaceted approach, including developing robust ethical guidelines, ensuring transparency and accountability, and involving diverse stakeholders in the development and deployment of AI systems. By doing so, we can harness the benefits of AI while mitigating its potential harms.
検証結果
英語プロンプトでの出力のほうが出力量く、かつ情報の網羅性も高かった。また、挙げられる例も具体性があり理解しやすい内容が出力された。
なぜこのような差が出たのか
日本語と英語での生成AIの出力結果の差について、言語構造とデータ量の観点から説明します。
言語構造の違い
1. 文法構造:
— 英語:
英語は主語-動詞-目的語(SVO)構造を持つため、文章が直線的で論理的に構成されています。この構造は、生成AIが文章を解析しやすく意図を明確に伝えることができます。
— 日本語:
日本語は主語-目的語-動詞(SOV)構造を持ち、主語や目的語が省略されることが多いです。このため、文の文脈やニュアンスを理解するのが難しく、生成AIが正確な出力を生成するのが難しくなることがあります。
2. 曖昧さと省略:
— 英語:
英語は比較的具体的で直接的な表現が好まれ、曖昧さを避ける傾向があります。このため、意図が明確に伝わりやすいと言えます。英語は具体的で直接的な表現が多く曖昧さが少ないです。生成AIはこれを活用して、明確で詳細な情報を生成しやすいです。
—日本語:
日本語はしばしば言葉を省略することが多く、曖昧な表現が多用されます。これはコミュニケーションにおいて柔軟性を持たせる一方で、意図が伝わりにくい場合もあります。そのため、生成AIが意図を正確に捉えるのが難しいことがあります。これが出力結果の質に影響します。
3. 敬語とニュアンス:
— 英語:
英語には敬語のような体系的な言い回しはありませんが、フォーマルな表現やカジュアルな表現を使い分けることがあります。ただし、日本語ほどの細かい階層はありません。
— 日本語:
日本語には敬語(尊敬語、謙譲語、丁寧語)があり、相手や状況に応じて使い分ける必要があります。このため、言葉の選び方が非常に重要であり、微妙なニュアンスを伝えることができます。
学習データ量の違い
1. データの豊富さ:
— 英語:
インターネット上の情報の多くが英語であり、生成AIは大量の英語データを基に学習しています。これにより、生成AIは多様で詳細な情報を提供する能力が高まります。
—日本語:
日本語のデータ量は英語に比べて少なく、特に専門的な情報や技術的なドキュメントの量が限られています。このため、生成AIが提供できる情報の質や量が英語に比べて劣ることがあります。
2. オープンデータセット:
— 英語:
英語には豊富なオープンデータセットがあり、これを基にした学習が進んでいます。英語のWikipediaやCommon Crawlなどがその例です。
— 日本語:
日本語のオープンデータセットは限られており、特に大規模なテキストデータは少ないです。このため、生成AIが学習するデータが少なくなり、出力結果に影響を与えます。
Wikipediaを例に挙げると、
英語版Wikipediaは約6,500万の記事があります。対して日本語版Wikipediaは約1,300万の記事で、英語版に比べると約5分の1の規模です。
出力結果の違い
1. 質の違い:
—英語:
豊富なデータ量と明確な文法構造により、英語の生成AIは文法的に正確で詳細な出力を生成する能力があります。特に技術的な内容や専門的な情報に関しては高い精度が期待できます。
— 日本語:
データ量の制約と文法構造の複雑さから、日本語の生成AIは文法的な間違いや自然でない表現が出ることがあります。また、詳細な情報や専門的な内容については、英語に比べて精度が劣ることがあります。
2. 量の違い:
— 英語:
英語の生成AIは豊富なデータに基づいているため、より多くの情報を提供し、特定のトピックに対する深掘りが可能です。
— 日本語:
日本語の生成AIはデータ量の制約から、提供できる情報の深さや広さが限られることがあります。特定のトピックに関する詳細な情報を生成する際に、データ不足が影響します。
結論
言語構造の違い
英語は直線的で明確な文法構造を持ち、生成AIが解析しやすいため、質の高い出力を生成しやすい。日本語は文法構造が複雑で曖昧さが多いため、意図を正確に伝えるのが難しい。
学習データ量の違い
英語は大量のデータを基に学習しているため、質・量ともに優れた出力を生成できる。日本語はデータ量が限られているため、出力の質や情報の深さに制約がある。
このような違いを理解することで、生成AIをより効果的に活用することができます。
(補足)
今回はコンテンツ生成検証を行いましたが、同様にコード生成(Python)も日本語プロンプトと英語プロンプトで出力結果を比較してみました。プログラミングの出力については、メソッド名や変数の命名の違い等はありましたが、処理の大きな違いはありませんでした。
プログラムコードを生成する際には、日本語でも要件を明確にプロンプトに組み込めば英語プロンプトと同レベルの出力ができると思われます。
この記事が気に入ったらサポートをしてみませんか?